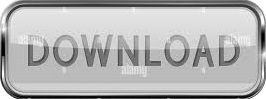
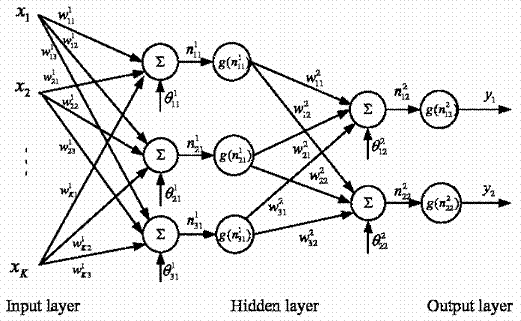
Figure 1: Illustration of existing and our regularization. The ability to overfit noise in training data often leads to poor performance. Deep CNNs are typically trained on large-scale data, the annotation on which are usually noisy. empirically demonstrated that common regularizers for neural networks such as weight decay, data augmentation and dropout, namely model regularizers, are less effective for improving the generation performance of deep convolutional neural networks (CNNs) trained on corrupted labels, an observation also confirmed by our study. Regularization is an effective approach to overcome overfitting. A recent study found deep networks are capable of memorizing the entire data even on corrupted labels, where some or all true labels are replaced with random labels. State-of-the-art deep networks have hundreds of layers and far more trainable model parameters than the number of samples on which they are trained.
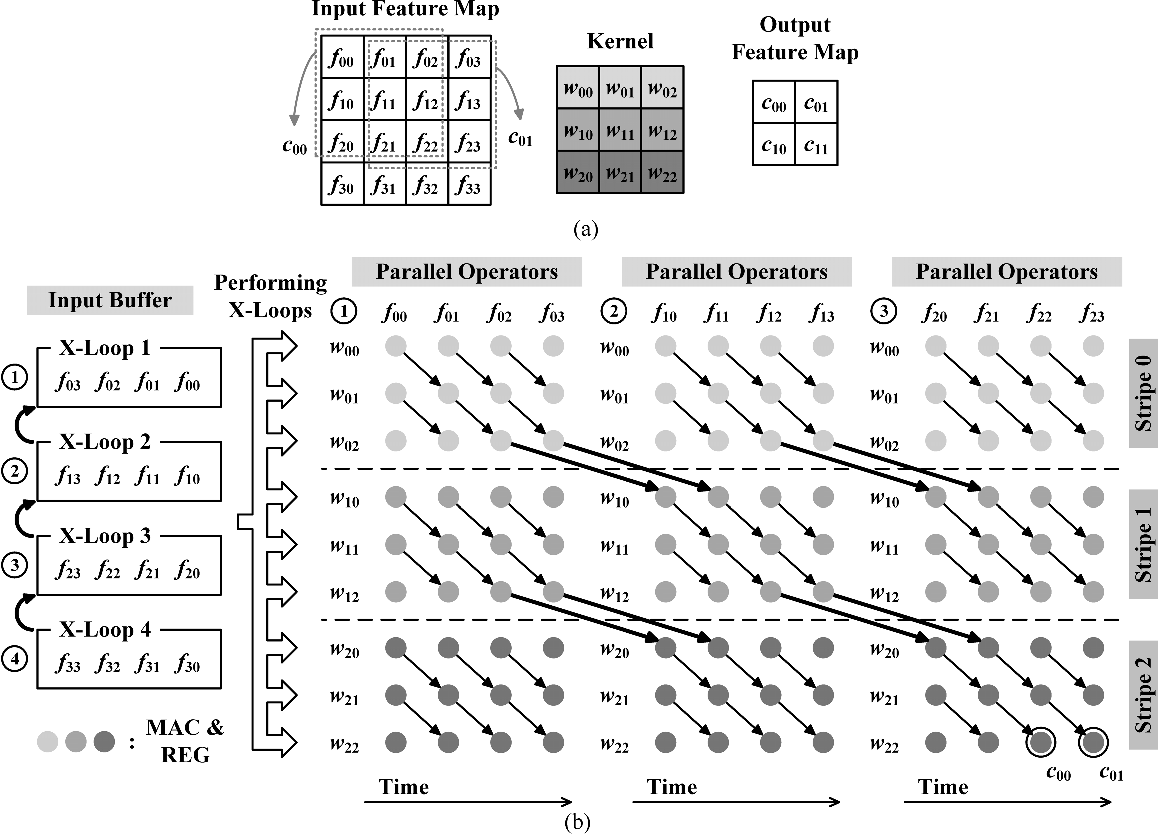
Deep neural networks have witnessed tremendous success in various vision tasks such as object recognition and detection.
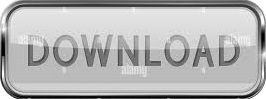